If data is not information, and information is not knowledge (according to a book skeptical about the prospects of the Internet and eCommerce in 1995), how can we make the leap from data to knowing what to do next? Although it may seem philosophical, this is also a very practical question for businesses seeking to use available data to make smart decisions. In this age of information overload, it’s easy to get lost along the way toward that end goal.
“Data” refers to facts, figures, or signals that are either unstructured or incomplete. For retailers and brands, those might be product inventory lists, price points, web traffic statistics, and so forth. Pieces of data must be processed and organized to become information, which in turn is combined with experience and understanding to arrive at knowledge. Realizing when and how to act upon knowledge is a strategic advantage in the commercial domain. This series of definitions mirrors the cycle that businesses go through to gain insight from the various information resources they access.
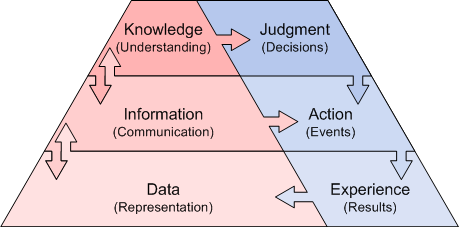
The data-information-knowledge pyramid, by Joe Gollner
From Stages of Knowledge to Steps in Data Processing
One of the most common procedures for dealing with data in many organizations is ETL, or Extract, Transform, Load:
- Extraction is obtaining data, usually from multiple sources, that may differ in quality and/or quantity. This is the raw data itself.
- Transformation means cleaning the data so that it conforms to certain standards. Once formatted and stored properly, data can be considered information.
- Loading the data to a destination, such as a data warehouse, allows it to be queried and analyzed, which leads to knowledge. The data may then be integrated with other data.
Action is the final step, the most significant, yet also the most challenging. The right course of action is subjectively determined by keeping your goals in mind along with the broader market context. For example, a retailer’s decision about whether or not to sell a new product should be based on not only its goal of boosting sales, but also an awareness of how the assortment it offers compares to that of others in the market.
This returns the focus to data, completing the ETL loop. A data-driven business constantly monitors key performance indicators for improvement opportunities and asks which metrics might be missing. Because of the globalization and digitization of shopping, retailers are now forced to keep track of competitors they may have never heard about, not just the well-known names. Tracking becomes increasingly difficult due to the amount of data points being compounded over time. Historical recordkeeping is the only way to detect trends in data.
In particular, Wiser is able to serve as a modern oracle for those interested in questions relevant to eCommerce intelligence. For example, let’s say you wanted to know, “How early did Easter dress promotions start?” To answer such a question, Wiser has developed advanced product information technologies that include web extraction, automated classification, and on-demand analytics.
Turning Online Product Information into Answers
So where do you start? Finding the data to gather. Retailer homepages, email messages, and social media posts are all inputs for Wiser’s extraction system. Converting those into data tables involves analyzing the titles, images, and prices displayed, among other product attributes. At that point, it can be determined which data contains a keyword of interest (“dress,” “Calvin Klein”) or has been identified with other characteristics (sales offered by Bloomingdale’s or Dillards). Further investigation can be done to surface relevant insights that may be presented according to customer requests.
“Garbage in, garbage out” remains a relevant maxim, since there can be discrepancies or pitfalls in the information presented. The products on a given website may not actually match what the shelf is supposed to consist of—when accessories are included in a grouping mainly for dresses, for instance. While in theory an item could belong to multiple product categories, in practice there should be a single correct classification to facilitate accuracy of measurement. Most eCommerce websites feature a defined taxonomy. It appears as a navigational menu that begins with top-level categories and progressively shows more specific ones. But different websites make different choices about how to classify their products in terms of granularity and arrangement (“Clothing” as a subcategory of “Women” or vice versa).
Wiser’s approach is to standardize the range of retailer taxonomies into one developed in-house, not considered superior, but simply more functional for our customers’ need to make apples-to-apples comparisons across the retail landscape. In fact, a taxonomy is a knowledge organization system that should evolve along with an enterprise. This uniform classification is a specialized form of data integration that is necessary as a foundation for all the insights provided. Without a high level of confidence about which product category a webpage or tweet belongs to, there couldn’t be any big-picture assertions about price or assortment.
Reaching the step of making an informed decision is only possible after a careful process of data collection, cleansing, and analysis. However, even that is no guarantee of results; as in the stock market, past performance is not a perfect predictor of the future.
The key for businesses committed to this strategic data-driven approach is investment in expertise and tools that support sound judgment in the long term. Learning to manage the data pipeline and associated systems comes at a cost, but it is well worth the potential benefits in growth and value.
Contributing writer: Eric Chuk
1 Comment